In today’s rapidly evolving digital landscape, the demand for skilled professionals in applied machine learning has surged dramatically. As industries embrace automation and data-driven decision-making, the need for individuals adept in machine learning techniques has become paramount. In this comprehensive review, we delve into the myriad benefits and opportunities awaiting those considering a career in applied machine learning.
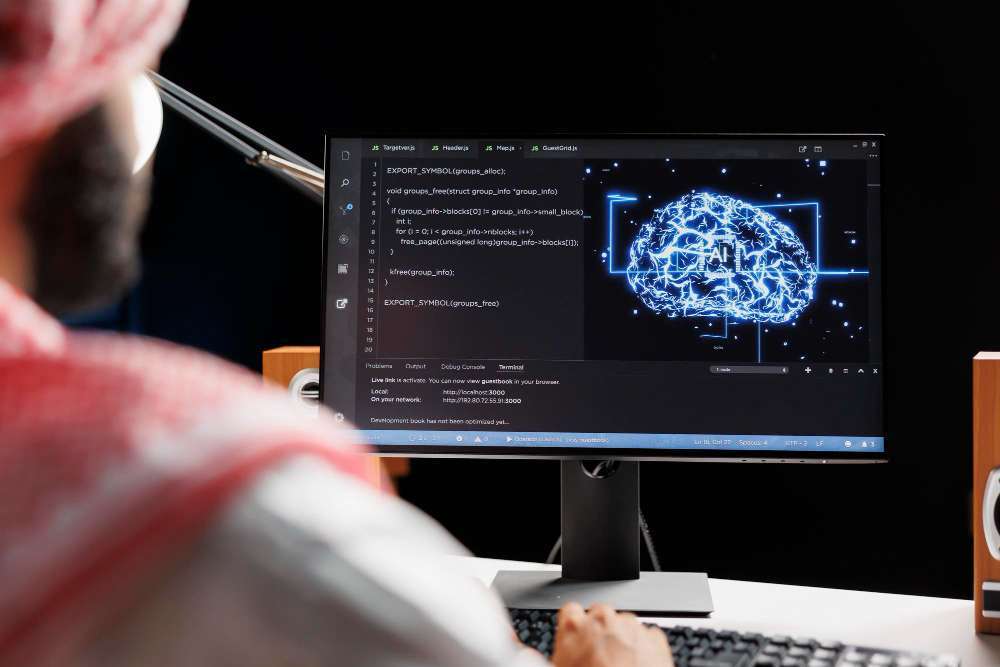
Job Market Trends
The job market for applied machine learning professionals is witnessing unprecedented growth, fueled by advancements in technology and the proliferation of data. According to recent studies, the demand for machine learning engineers, data scientists, and AI specialists is projected to continue rising exponentially over the next decade. Industries ranging from healthcare and finance to e-commerce and manufacturing are actively seeking professionals with expertise in machine learning to drive innovation and gain a competitive edge.
Education and Training Requirements
Embarking on a career in applied machine learning typically requires a solid foundation in computer science, mathematics, and statistics. While a bachelor’s degree in a related field is often sufficient to enter the field, many employers prefer candidates with advanced degrees such as master’s or doctoral degrees. Additionally, acquiring specialized training through online courses, bootcamps, or certifications can significantly enhance one’s employability and earning potential in this field.
Salary and Benefits
The remuneration for professionals in applied machine learning is notably lucrative, reflecting the high demand for their skills and expertise. Salaries vary depending on factors such as experience, education, location, and industry. On average, machine learning engineers and data scientists command six-figure salaries, with ample opportunities for bonuses, stock options, and other incentives. Moreover, many employers offer attractive benefits packages, including health insurance, retirement plans, and flexible work arrangements, further enriching the overall compensation package.
Work-Life Balance
Contrary to popular misconceptions, professionals in applied machine learning enjoy a favorable work-life balance, thanks to the flexibility afforded by remote work and flexible scheduling options. While the nature of the work may involve occasional long hours and tight deadlines, many employers prioritize employee well-being and promote a healthy work-life balance culture. Additionally, advancements in collaboration tools and communication technologies enable seamless remote work arrangements, allowing professionals to maintain productivity while accommodating personal commitments.
Job Stability
The demand for skilled professionals in applied machine learning shows no signs of abating, ensuring robust job stability in the years to come. As businesses across industries continue to leverage data-driven insights to drive growth and innovation, the need for individuals proficient in machine learning algorithms and techniques will only intensify. Furthermore, the interdisciplinary nature of machine learning equips professionals with transferable skills that remain relevant across various domains, further enhancing their long-term employability and job security.
Networking Opportunities
Networking plays a pivotal role in advancing one’s career in applied machine learning, offering valuable opportunities for learning, collaboration, and professional growth. Engaging with industry peers, attending conferences, and participating in online communities can foster meaningful connections and open doors to new career opportunities. Moreover, networking enables professionals to stay abreast of the latest trends, developments, and job openings in the field, positioning them for success in a competitive job market.
Industry Specifics
Applied machine learning finds applications across a diverse array of industries, each presenting unique opportunities and challenges for professionals in the field. In healthcare, machine learning algorithms are revolutionizing diagnostics, treatment planning, and patient care, leading to improved outcomes and reduced costs. Similarly, in finance, machine learning models are employed for fraud detection, risk assessment, and algorithmic trading, enhancing operational efficiency and mitigating financial risks. From marketing and retail to cybersecurity and autonomous vehicles, the potential applications of machine learning are virtually limitless, offering ample opportunities for professionals to make meaningful contributions and drive innovation.
Skills Gap Analysis
While the demand for professionals in applied machine learning is soaring, there exists a significant skills gap that poses challenges for employers and job seekers alike. Employers often struggle to find candidates with the requisite skills and expertise to fill critical roles, leading to talent shortages and prolonged recruitment cycles. Conversely, aspiring professionals face the challenge of acquiring specialized skills and staying abreast of rapidly evolving technologies and methodologies. Bridging the skills gap requires concerted efforts from academia, industry, and policymakers to develop comprehensive training programs, promote lifelong learning, and foster a culture of innovation and collaboration.
Future Trends and Technology
The future of applied machine learning holds immense promise, driven by advancements in technology and the growing sophistication of machine learning algorithms. Emerging trends such as deep learning, natural language processing, and reinforcement learning are poised to revolutionize industries and unlock new opportunities for innovation and growth. Moreover, the convergence of machine learning with other emerging technologies such as blockchain, Internet of Things (IoT), and augmented reality (AR) is opening up new frontiers for exploration and experimentation. By staying abreast of these trends and embracing lifelong learning, professionals can position themselves for success in an ever-changing landscape.
Risk Assessment
While the prospects for a career in applied machine learning are undeniably promising, it is essential for aspiring professionals to conduct a thorough risk assessment and weigh the potential challenges and opportunities associated with the field. Risks such as job automation, skill obsolescence, and ethical considerations should be carefully considered and addressed through proactive measures such as continuous learning, skill diversification, and ethical awareness training. By taking a proactive approach to risk management and embracing uncertainty, professionals can navigate the complexities of the field and thrive in an increasingly competitive and dynamic environment.
Additional Resources:
- Unlocking the Future: A Career in 3D Printing Applications in Product Design
- Unlocking Your Potential: Exploring Career Opportunities in Personal Development and Self-Improvement
- Master Your Wealth: Exploring Careers in Personal Finance and Investment
- Building Wealth, Building Futures: Exploring Careers in Personal Finance and Wealth Management
- Navigating the Cosmos: Exploring Careers in Planetary Science and Exploration
- Shaping the Future: A Career in Education
- Behind the Scenes: A Career in Film Studies
- Mastering Money: A Career in Financial Literacy and Money Management
- Streamlining Success: The Advantages of Pursuing a Course in Industrial Automation
- Introduction to Accounting Principles
Table of Contents
Categories:
Latest Posts:
FAQ’s
1. What is applied machine learning?
Applied machine learning involves the use of machine learning techniques and algorithms to solve real-world problems across various industries, including finance, healthcare, e-commerce, and more. It focuses on the practical implementation of these techniques to develop predictive models, improve processes, and create intelligent systems.
2. What educational background is needed to pursue a career in applied machine learning?
A strong educational background in computer science, data science, statistics, or a related field is essential. Degrees such as a Bachelor’s or Master’s in these areas, often supplemented by specialized courses or certifications in machine learning and artificial intelligence, are commonly pursued.
3. What skills are essential for success in applied machine learning?
Key skills include proficiency in programming languages (like Python and R), a solid understanding of algorithms and data structures, knowledge of statistical methods, experience with machine learning frameworks (such as TensorFlow and PyTorch), and strong problem-solving abilities. Familiarity with data preprocessing, model evaluation, and deployment is also important.
4. How can one gain practical experience in applied machine learning?
Practical experience can be gained through internships, research projects, and hands-on practice with datasets. Participating in machine learning competitions (like Kaggle), contributing to open-source projects, and completing industry-specific projects during coursework also provide valuable experience.
5. What are some common applications of machine learning in various industries?
Machine learning is applied in numerous fields, including finance (fraud detection, risk assessment), healthcare (diagnostics, personalized medicine), e-commerce (recommendation systems, customer segmentation), and autonomous systems (self-driving cars, robotics), among others.